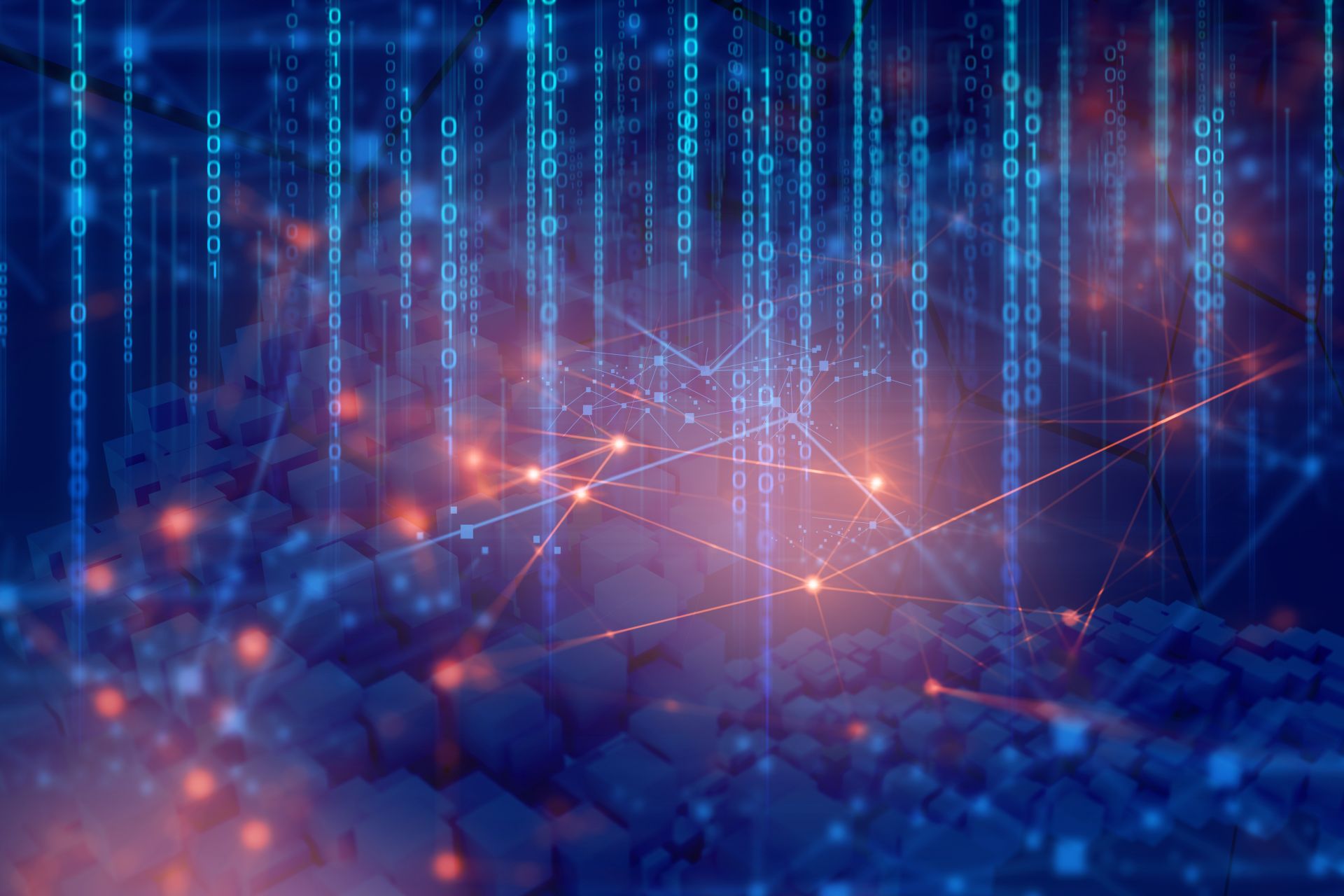
Research
Discover how our researchers are addressing the challenges that exist at the intersection of information, technology and society.
Research in IST focuses on systems-level thinking, higher conceptualization of global problems, and interdisciplinary connectivity. We explore innovative solutions for dealing with the complex and emerging problems that are changing the way we think, live, and engage with the world. Our faculty, students, and scholars represent the best Penn State has to offer.
$79.7M
External research funding credited to IST faculty
497
External research grants credited to IST faculty
117
Funding agencies supporting IST research
$11.5M
Research expenditures (FY24)
$1M+
Seed grants and matching funds awarded by IST
47
Industry partnerships
112
Active research grants
24
IST faculty received NSF CAREER Awards
$6M+
NSF CAREER Award funding
Since 2015
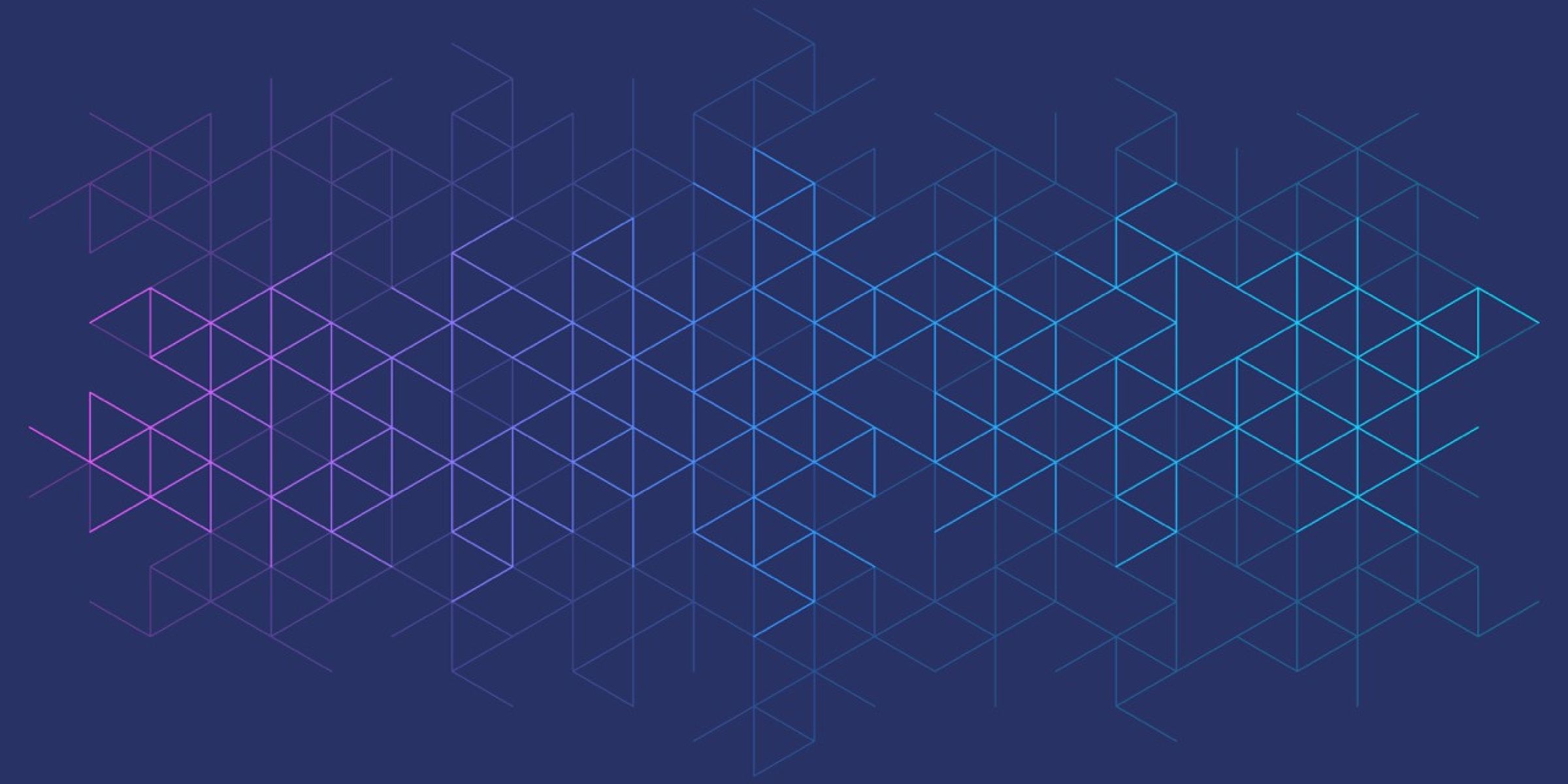
Research or Regress
At Penn State, researchers are solving real problems that impact the health, safety, and quality of life of people across the Commonwealth, the nation, and around the world.
For decades, federal support for research has fueled innovation that makes our country safer, our industries more competitive, and our economy stronger. Recent federal funding cuts threaten this progress.
Learn more about the implications of federal funding cuts to your future.
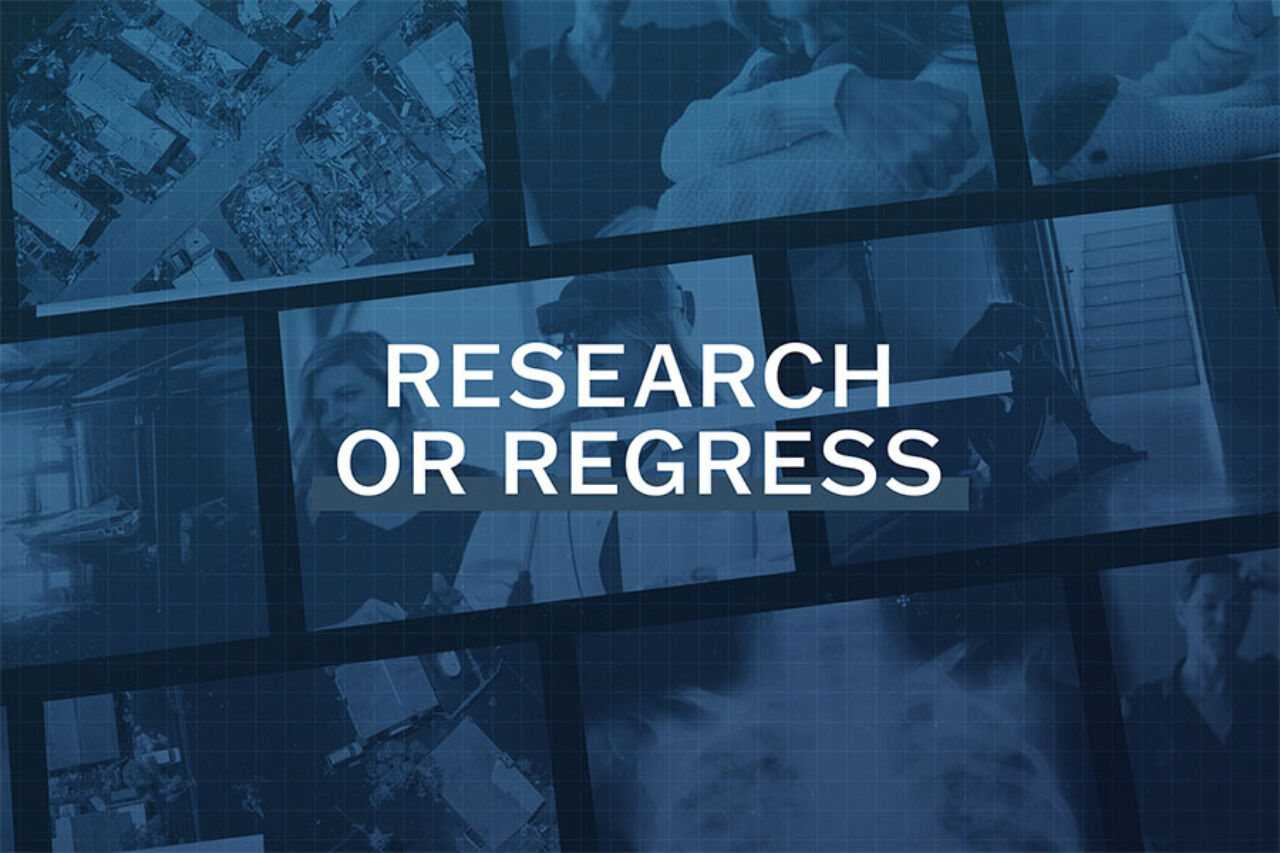
Research News
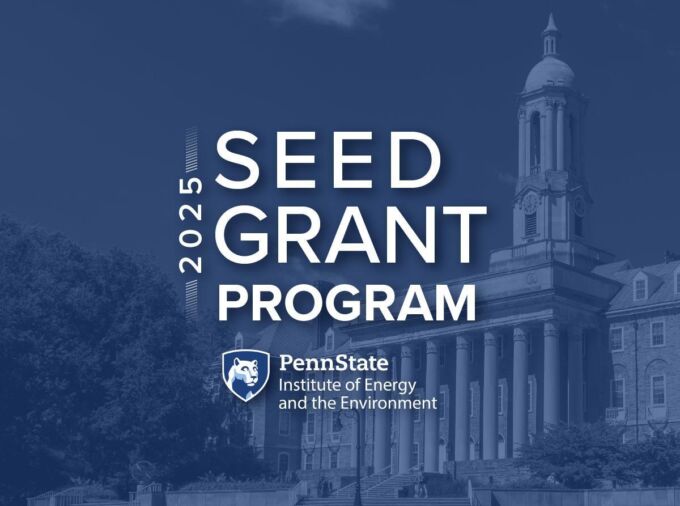
June 24, 2025
Ten interdisciplinary research teams awarded IEE seed grants
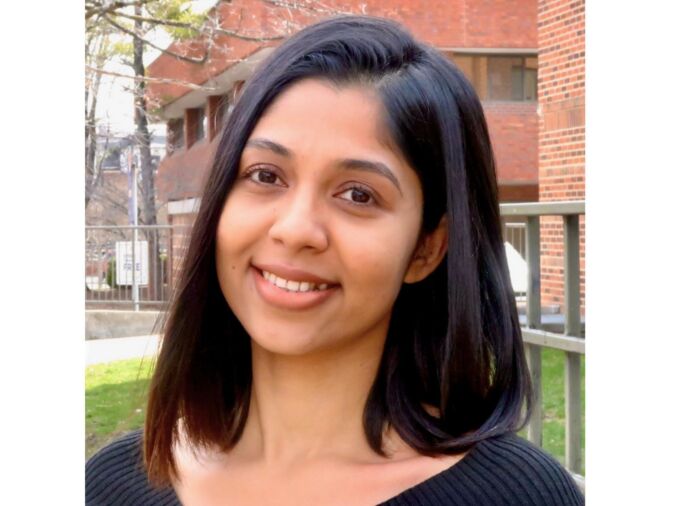
June 30, 2025
Q&A: Improving how blind and low-vision people navigate deepfakes
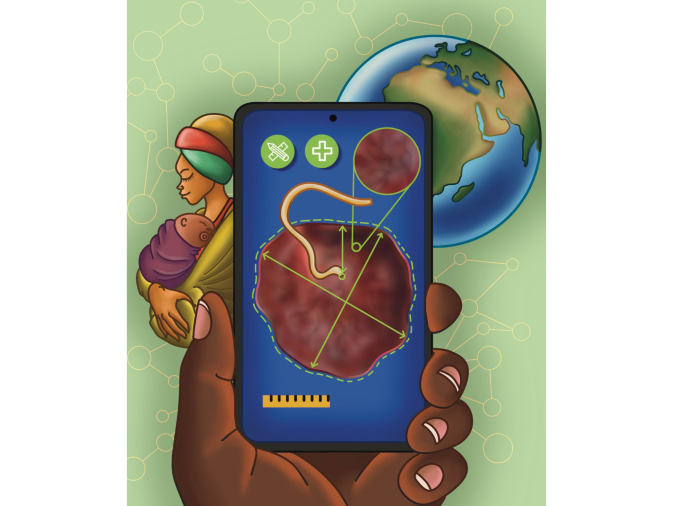
December 17, 2024
Placenta assessment tool aims to improve neonatal, maternal care
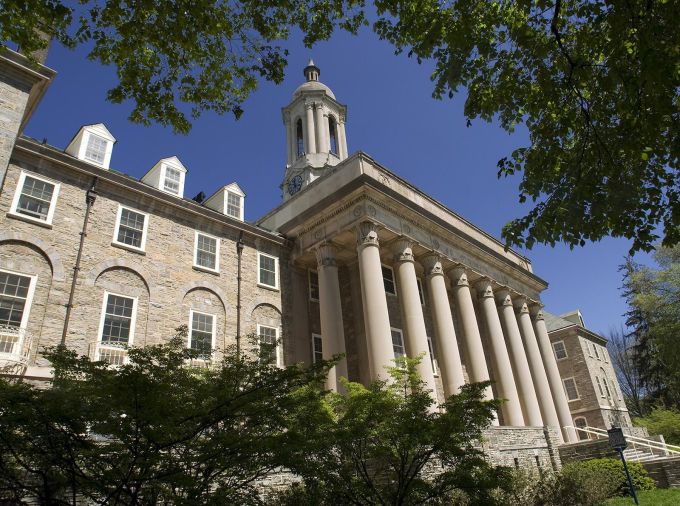
April 04, 2025